Python Slots Performance
Antonin Bruneau is a Solution Engineer at StreamSets, based in Paris, France. He recently created a fun demo system showing how the StreamSets DataOps Platform can collect, ingest, and transform IoT data. Over to you, Antonin…
Traditional product blog posts explain how to solve complex problems you might find in your daily job, but today I want to show you how to have a fun time with StreamSets.
Slots are very useful for library calls to eliminate the “named method dispatch” when making function calls. This is mentioned in the SWIG documentation. For high performance libraries that want to reduce function overhead for commonly called functions using slots is much faster. Now this may not be directly related to the OPs question. When a signal connected to a handler.slot is emitted. The script times how long it takes to emit a million signals, does this a 1000 times and averages. The difference is not significant: the gain is 0% in the above capture, and a couple separate run showed around 2-4%. Python comes with the cProfile module to help evaluate performance. It not only gives the total running time, it also times each function separately. It then tells you how many times each function was called, which makes it easy to determine where you should make optimizations. Here's what a sample analysis by cProfile looks like. Print('Welcome to the Slot Machine Simulator: You'll start with $50. You'll be asked if you want to play. Answer with yes/no. You can also use y/n: No case sensitivity in your answer. For example you can answer with YEs, yEs, Y, nO, N. To win you must get one of the following combinations: BARtBARtBARttpayst$250: BELLtBELLtBELL/BAR.
Who doesn’t remember having great fun racing slot cars, competing with your best friend, and epic car crashes?
In this blog post, I’ll show you how to add sensors to your race track with a Raspberry Pi, and then collect metrics in real-time and compute statistics about your races, using StreamSets Data Collector and StreamSets Transformer.
The Electronic Part
To measure your race performance, we are going to add sensors to the start/finish line of the race track, and some buttons to control the game. We will use a Raspberry Pi to collect the measurements and output the events to a file.
Details about the components, schematics, and code for the Raspberry can be found here: https://github.com/abruneau/hacking_electric_race_track.
Event Collection
Reading Data from the Raspberry Pi
On the Raspberry Pi, a Python application reads the sensor and button events and writes them to a text file. Each line in the file is composed of a timestamp and an event number. To have a minimum impact on the device, we will use StreamSets Data Collector Edge to read the file and send the events to a Kafka topic.
Converting Raw Data to Game Information
In this second part of event collection, we will parse the raw data and store the results in MySQL tables. Here is the schema I used:
And here is the pipeline to read Kafka and write data to MySQL:
From Kafka, we receive a series of timestamps and event numbers:
- 0: race starts
- 1: sensor 1
- 2: sensor 2
- 3: race stops
- 4: reset race
A Stream Selector (‘dispatch events’ in the pipeline shown above) dispatches the events to different routes.
The start route (event 0) will retrieve the newly created game (see below how we create it with the API) and set the start time for it.
The sensor route (event 1 and 2) uses Redis to cache each sensor event timestamp and retrieve the previous one if it exists. If the sensor has been triggered before, we can calculate the duration of the lap and update the Laps table.
The stop route (event 3) retrieves the current game and updates the game table with the finish time. It also triggers two other pipelines. One Data Collector pipeline that will update the winner table, and a Transformer pipeline I will explain later.
The last one, the reset route (event 4), gets the current game and resets it by deleting all associated laps, resets the winner table, Redis, and start/stop time of the game.
The pipeline responsible for setting the winner is very simple. It uses a SQL query to find the user based on the game Id we pass in params and stores the results in the winners’ table. A Pipeline finisher stops it when the winner has been set and resets the origin.
Game Statistics
To add some more competition, a Transformer pipeline is used to compute statistics about the games.
This pipeline joins the players table with the laps table to rank players on their fastest lap and their fastest overall race. You could easily compute more statistics like average lap/race time, or player stats to measure progress. It is up to you to get creative.
This pipeline is triggered by the stop route of the Data Collector pipeline.
Exposing the Data
Now that we can collect the race track data, comes the question of exposing them. I could have chosen a traditional BI tool and plugged it into the database directly but I wanted something light that I could customize easily. So I created a small web app in Angular that queries an API to collect data.
I built the API using a Data Collector microservice pipeline.
This is a special type of pipeline that has a REST Service origin as input and sends back an HTTP response. I used an HTTP Router to route the event depending on the URL path. To retrieve the data, I used a series of JDBC Lookup processors, and Expression Evaluators to format the response. This blog post explains more about microservice pipelines, and contains a link to a tutorial.
Wrap Up
By using StreamSets, we were able to collect data on an IoT device, stream the events from Kafka, populate a database, compute statistics leveraging Spark, and expose data through an API.
Now it is your turn! Play it, hack it, share it!!
This GitHub repository contains a docker-compose file with StreamSets Data Collector and StreamSets Transformer, local Spark, Kafka (Lenses), and the Web UI: https://github.com/streamsets/slot_car_demo
Categories
Products
The Question :
What is the purpose of __slots__
in Python — especially with respect to when I would want to use it, and when not?
The Answer 1
TLDR:
The special attribute __slots__
allows you to explicitly state which instance attributes you expect your object instances to have, with the expected results:
- faster attribute access.
- space savings in memory.
The space savings is from
- Storing value references in slots instead of
__dict__
. - Denying
__dict__
and__weakref__
creation if parent classes deny them and you declare__slots__
.
Quick Caveats
Small caveat, you should only declare a particular slot one time in an inheritance tree. For example:
Python doesn’t object when you get this wrong (it probably should), problems might not otherwise manifest, but your objects will take up more space than they otherwise should. Python 3.8:
This is because the Base’s slot descriptor has a slot separate from the Wrong’s. This shouldn’t usually come up, but it could:
The biggest caveat is for multiple inheritance – multiple “parent classes with nonempty slots” cannot be combined.
To accommodate this restriction, follow best practices: Factor out all but one or all parents’ abstraction which their concrete class respectively and your new concrete class collectively will inherit from – giving the abstraction(s) empty slots (just like abstract base classes in the standard library).
See section on multiple inheritance below for an example.
Requirements:
To have attributes named in
__slots__
to actually be stored in slots instead of a__dict__
, a class must inherit fromobject
.To prevent the creation of a
__dict__
, you must inherit fromobject
and all classes in the inheritance must declare__slots__
and none of them can have a'__dict__'
entry.
There are a lot of details if you wish to keep reading.
Why use __slots__
: Faster attribute access.
The creator of Python, Guido van Rossum, states that he actually created __slots__
for faster attribute access.
It is trivial to demonstrate measurably significant faster access:
and
The slotted access is almost 30% faster in Python 3.5 on Ubuntu.
In Python 2 on Windows I have measured it about 15% faster.
Why use __slots__
: Memory Savings
Another purpose of __slots__
is to reduce the space in memory that each object instance takes up.
My own contribution to the documentation clearly states the reasons behind this:
The space saved over using __dict__
can be significant.
SQLAlchemy attributes a lot of memory savings to __slots__
.
To verify this, using the Anaconda distribution of Python 2.7 on Ubuntu Linux, with guppy.hpy
(aka heapy) and sys.getsizeof
, the size of a class instance without __slots__
declared, and nothing else, is 64 bytes. That does not include the __dict__
. Thank you Python for lazy evaluation again, the __dict__
is apparently not called into existence until it is referenced, but classes without data are usually useless. When called into existence, the __dict__
attribute is a minimum of 280 bytes additionally.
In contrast, a class instance with __slots__
declared to be ()
(no data) is only 16 bytes, and 56 total bytes with one item in slots, 64 with two.
For 64 bit Python, I illustrate the memory consumption in bytes in Python 2.7 and 3.6, for __slots__
and __dict__
(no slots defined) for each point where the dict grows in 3.6 (except for 0, 1, and 2 attributes):
So, in spite of smaller dicts in Python 3, we see how nicely __slots__
scale for instances to save us memory, and that is a major reason you would want to use __slots__
.
Just for completeness of my notes, note that there is a one-time cost per slot in the class’s namespace of 64 bytes in Python 2, and 72 bytes in Python 3, because slots use data descriptors like properties, called “members”.
Demonstration of __slots__
:
To deny the creation of a __dict__
, you must subclass object
:
now:
Or subclass another class that defines __slots__

and now:
but:
To allow __dict__
creation while subclassing slotted objects, just add '__dict__'
to the __slots__
(note that slots are ordered, and you shouldn’t repeat slots that are already in parent classes):
and
Or you don’t even need to declare __slots__
in your subclass, and you will still use slots from the parents, but not restrict the creation of a __dict__
:
And:
However, __slots__
may cause problems for multiple inheritance:
Because creating a child class from parents with both non-empty slots fails:
If you run into this problem, You could just remove __slots__
from the parents, or if you have control of the parents, give them empty slots, or refactor to abstractions:
Add '__dict__'
to __slots__
to get dynamic assignment:
and now:
So with '__dict__'
in slots we lose some of the size benefits with the upside of having dynamic assignment and still having slots for the names we do expect.
When you inherit from an object that isn’t slotted, you get the same sort of semantics when you use __slots__
– names that are in __slots__
point to slotted values, while any other values are put in the instance’s __dict__
.
Avoiding __slots__
because you want to be able to add attributes on the fly is actually not a good reason – just add '__dict__'
to your __slots__
if this is required.
You can similarly add __weakref__
to __slots__
explicitly if you need that feature.
Set to empty tuple when subclassing a namedtuple:
The namedtuple builtin make immutable instances that are very lightweight (essentially, the size of tuples) but to get the benefits, you need to do it yourself if you subclass them:
usage:
And trying to assign an unexpected attribute raises an AttributeError
because we have prevented the creation of __dict__
:
You can allow __dict__
creation by leaving off __slots__ = ()
, but you can’t use non-empty __slots__
with subtypes of tuple.

Biggest Caveat: Multiple inheritance
Even when non-empty slots are the same for multiple parents, they cannot be used together:
Using an empty __slots__
in the parent seems to provide the most flexibility, allowing the child to choose to prevent or allow (by adding '__dict__'
to get dynamic assignment, see section above) the creation of a __dict__
:
You don’t have to have slots – so if you add them, and remove them later, it shouldn’t cause any problems.
Going out on a limb here: If you’re composing mixins or using abstract base classes, which aren’t intended to be instantiated, an empty __slots__
in those parents seems to be the best way to go in terms of flexibility for subclassers.
To demonstrate, first, let’s create a class with code we’d like to use under multiple inheritance
We could use the above directly by inheriting and declaring the expected slots:
But we don’t care about that, that’s trivial single inheritance, we need another class we might also inherit from, maybe with a noisy attribute:
Now if both bases had nonempty slots, we couldn’t do the below. (In fact, if we wanted, we could have given AbstractBase
nonempty slots a and b, and left them out of the below declaration – leaving them in would be wrong):
And now we have functionality from both via multiple inheritance, and can still deny __dict__
and __weakref__
instantiation:
Other cases to avoid slots:
- Avoid them when you want to perform
__class__
assignment with another class that doesn’t have them (and you can’t add them) unless the slot layouts are identical. (I am very interested in learning who is doing this and why.) - Avoid them if you want to subclass variable length builtins like long, tuple, or str, and you want to add attributes to them.
- Avoid them if you insist on providing default values via class attributes for instance variables.
You may be able to tease out further caveats from the rest of the __slots__
documentation (the 3.7 dev docs are the most current), which I have made significant recent contributions to.
Critiques of other answers
The current top answers cite outdated information and are quite hand-wavy and miss the mark in some important ways.
Do not “only use __slots__
when instantiating lots of objects”
I quote:
“You would want to use __slots__
if you are going to instantiate a lot (hundreds, thousands) of objects of the same class.”
Abstract Base Classes, for example, from the collections
module, are not instantiated, yet __slots__
are declared for them.
Why?
If a user wishes to deny __dict__
or __weakref__
creation, those things must not be available in the parent classes.
__slots__
contributes to reusability when creating interfaces or mixins.
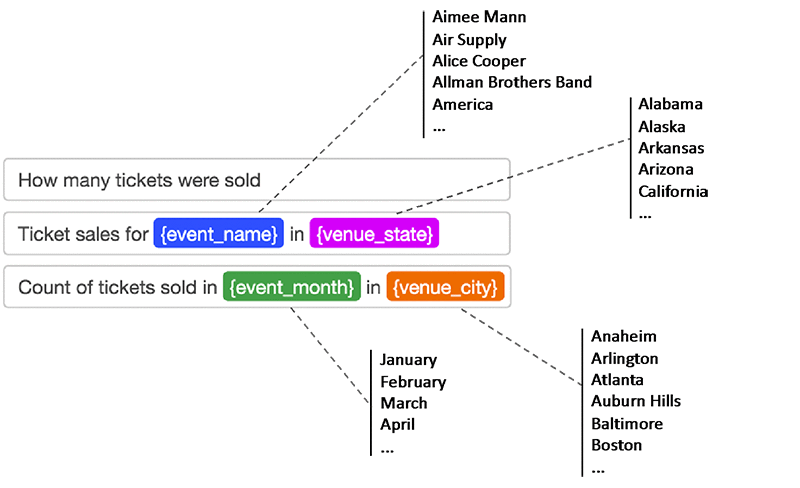
It is true that many Python users aren’t writing for reusability, but when you are, having the option to deny unnecessary space usage is valuable.
__slots__
doesn’t break pickling
When pickling a slotted object, you may find it complains with a misleading TypeError
:
This is actually incorrect. This message comes from the oldest protocol, which is the default. You can select the latest protocol with the -1
argument. In Python 2.7 this would be 2
(which was introduced in 2.3), and in 3.6 it is 4
.
in Python 2.7:
Python Slot Machine Code
in Python 3.6
So I would keep this in mind, as it is a solved problem.
Critique of the (until Oct 2, 2016) accepted answer
The first paragraph is half short explanation, half predictive. Here’s the only part that actually answers the question
The proper use of __slots__
is to save space in objects. Instead of having a dynamic dict that allows adding attributes to objects at anytime, there is a static structure which does not allow additions after creation. This saves the overhead of one dict for every object that uses slots
The second half is wishful thinking, and off the mark:
While this is sometimes a useful optimization, it would be completely unnecessary if the Python interpreter was dynamic enough so that it would only require the dict when there actually were additions to the object.
Python actually does something similar to this, only creating the __dict__
when it is accessed, but creating lots of objects with no data is fairly ridiculous.
The second paragraph oversimplifies and misses actual reasons to avoid __slots__
. The below is not a real reason to avoid slots (for actual reasons, see the rest of my answer above.):
They change the behavior of the objects that have slots in a way that can be abused by control freaks and static typing weenies.
It then goes on to discuss other ways of accomplishing that perverse goal with Python, not discussing anything to do with __slots__
.
The third paragraph is more wishful thinking. Together it is mostly off-the-mark content that the answerer didn’t even author and contributes to ammunition for critics of the site.
Create some normal objects and slotted objects:
Instantiate a million of them:
Inspect with guppy.hpy().heap()
:
Access the regular objects and their __dict__
and inspect again:
This is consistent with the history of Python, from Unifying types and classes in Python 2.2
If you subclass a built-in type, extra space is automatically added to the instances to accomodate __dict__
and __weakrefs__
. (The __dict__
is not initialized until you use it though, so you shouldn’t worry about the space occupied by an empty dictionary for each instance you create.) If you don’t need this extra space, you can add the phrase “__slots__ = []
” to your class.
The Answer 2
Quoting Jacob Hallen:
The proper use of __slots__
is to save space in objects. Instead of having a dynamic dict that allows adding attributes to objects at anytime, there is a static structure which does not allow additions after creation. [This use of __slots__
eliminates the overhead of one dict for every object.] While this is sometimes a useful optimization, it would be completely unnecessary if the Python interpreter was dynamic enough so that it would only require the dict when there actually were additions to the object.
Unfortunately there is a side effect to slots. They change the behavior of the objects that have slots in a way that can be abused by control freaks and static typing weenies. This is bad, because the control freaks should be abusing the metaclasses and the static typing weenies should be abusing decorators, since in Python, there should be only one obvious way of doing something.
Making CPython smart enough to handle saving space without __slots__
is a major undertaking, which is probably why it is not on the list of changes for P3k (yet).
The Answer 3
You would want to use __slots__
if you are going to instantiate a lot (hundreds, thousands) of objects of the same class. __slots__
only exists as a memory optimization tool.
It’s highly discouraged to use __slots__
for constraining attribute creation.
Pickling objects with __slots__
won’t work with the default (oldest) pickle protocol; it’s necessary to specify a later version.
High Performance Python
Some other introspection features of python may also be adversely affected.
The Answer 4
Each python object has a __dict__
atttribute which is a dictionary containing all other attributes. e.g. when you type self.attr
python is actually doing self.__dict__['attr']
. As you can imagine using a dictionary to store attribute takes some extra space & time for accessing it.
However, when you use __slots__
, any object created for that class won’t have a __dict__
attribute. Instead, all attribute access is done directly via pointers.
So if want a C style structure rather than a full fledged class you can use __slots__
for compacting size of the objects & reducing attribute access time. A good example is a Point class containing attributes x & y. If you are going to have a lot of points, you can try using __slots__
in order to conserve some memory.
The Answer 5
In addition to the other answers, here is an example of using __slots__
:
Slot Machine In Python
So, to implement __slots__
, it only takes an extra line (and making your class a new-style class if it isn’t already). This way you can reduce the memory footprint of those classes 5-fold, at the expense of having to write custom pickle code, if and when that becomes necessary.
The Answer 6
Slots are very useful for library calls to eliminate the “named method dispatch” when making function calls. This is mentioned in the SWIG documentation. For high performance libraries that want to reduce function overhead for commonly called functions using slots is much faster.
Now this may not be directly related to the OPs question. It is related more to building extensions than it does to using the slots syntax on an object. But it does help complete the picture for the usage of slots and some of the reasoning behind them.
The Answer 7
An attribute of a class instance has 3 properties: the instance, the name of the attribute, and the value of the attribute.
In regular attribute access, the instance acts as a dictionary and the name of the attribute acts as the key in that dictionary looking up value.
instance(attribute) –> value
In __slots__ access, the name of the attribute acts as the dictionary and the instance acts as the key in the dictionary looking up value.
attribute(instance) –> value
In flyweight pattern, the name of the attribute acts as the dictionary and the value acts as the key in that dictionary looking up the instance.
attribute(value) –> instance
The Answer 8
A very simple example of __slot__
attribute.
Problem: Without __slots__
If I don’t have __slot__
attribute in my class, I can add new attributes to my objects.
If you look at example above, you can see that obj1 and obj2 have their own x and y attributes and python has also created a dict
attribute for each object (obj1 and obj2).
Suppose if my class Test has thousands of such objects? Creating an additional attribute dict
for each object will cause lot of overhead (memory, computing power etc.) in my code.
Solution: With __slots__
Now in the following example my class Test contains __slots__
attribute. Now I can’t add new attributes to my objects (except attribute x
) and python doesn’t create a dict
attribute anymore. This eliminates overhead for each object, which can become significant if you have many objects.
The Answer 9
Another somewhat obscure use of __slots__
is to add attributes to an object proxy from the ProxyTypes package, formerly part of the PEAK project. Its ObjectWrapper
allows you to proxy another object, but intercept all interactions with the proxied object. It is not very commonly used (and no Python 3 support), but we have used it to implement a thread-safe blocking wrapper around an async implementation based on tornado that bounces all access to the proxied object through the ioloop, using thread-safe concurrent.Future
objects to synchronise and return results.
By default any attribute access to the proxy object will give you the result from the proxied object. If you need to add an attribute on the proxy object, __slots__
can be used.
The Answer 10
You have — essentially — no use for __slots__
.
For the time when you think you might need __slots__
, you actually want to use Lightweight or Flyweight design patterns. These are cases when you no longer want to use purely Python objects. Instead, you want a Python object-like wrapper around an array, struct, or numpy array.
The class-like wrapper has no attributes — it just provides methods that act on the underlying data. The methods can be reduced to class methods. Indeed, it could be reduced to just functions operating on the underlying array of data.
The Answer 11
The original question was about general use cases not only about memory.So it should be mentioned here that you also get better performance when instantiating large amounts of objects – interesting e.g. when parsing large documents into objects or from a database.
Here is a comparison of creating object trees with a million entries, using slots and without slots. As a reference also the performance when using plain dicts for the trees (Py2.7.10 on OSX):
Test classes (ident, appart from slots):
testcode, verbose mode: